How to get a job in Analytics at a big retailer
The trick isn't knowing everything, the trick is knowing what matters.
So, you’re about to enter an interview for that Analytics position in a big retailer. To get it, you have to demonstrate domain expertise and that’s why you’re here.
There are many ways to convey the idea you know about something.
In the short time of an interview, the bozos, the ones that don’t truly understand what they are talking about, will babble about whatever sexy retailer tech they’ve read in the news. Savvy interviewers, however will be on the lookout for people who master the fundamentals. In the case of retail analytics, that is the loyalty program.
Ask anyone what a loyalty program is and the answer will likely be something about keeping your customers loyal to your business. And yet, how many of us, who carry several different loyalty cards inside our wallets, are actually loyal to any loyalty program?
In reality, what we call loyalty programs are customer identification programs.
The ability to identify your customer is the cornerstone of any analytics program worth of its salt. It’s a capability largely underestimated by people with a digital background, where customer identification is as trivial as collecting one’s email or adding cookies to their device, but a major challenge to traditional brick and mortar retailers.
Customers are the North Star
In war, Roman generals knew they had to present their plans in its simplest possible form so that everyone in the army had a clear focus. The best companies do the same for their products: a single north star metric that best captures the core value their product delivers to their customers. This metric will be something specific to each industry - eCommerce companies care about conversion, messaging apps care about messages sent by user, mobile games care about average revenue per user.
Oh, but there are also the vanity metrics: things you can measure that don't matter. App downloads and registered users are the classical examples: naive executives commonly mistake these for indicators of growth and may never notice their apps were only used once and then uninstalled.
In traditional retail, the store is the product; visits are the vanity metric; and shoppers are the north star.
Without being able to identify customers, retailers have no way of saying whether or not they are losing their public to the competition. They only have visits and basket data.
Let’s imagine a scenario where we know every human needs only three grocery categories to survive: food, cleaning items and health care items. You have 2 baskets: one with cleaning and health care items and another just with food. Because you expect every person to buy three categories, there can be two explanations:
If each basket belongs to a different shopper, your customers are not loyal. The first one is getting their food somewhere else - most likely a competitor.
If both baskets are from the same shopper, that made visits in different days, what you have is a specific customer behavior
However, without customer identification there is no way to say whether you’re seeing your customer fly to the competition or just a specific shopping behavior. That’s why Classic Retail Analytics had retailers relying on imperfect proxies, such as customer panels, to infer what was happening to their business.
Enter the Commercial Equation
The rise of a customer ID is what sets the current Retail Analytics (also known as Advanced Analytics or Customer Analytics) apart from the Classical Analytics we had through most of the last century. With a loyalty program in place and the customer properly identified, retailers can figure out their north star: how many customers they actually have and how different customer segments differ from each other.
This gave rise to the following framework:
sales are broken into “sales by customer” and “total customers”
“sales by customer” are broken into “frequency” and “sales by basket”
finally, “sales by basket” is broken into “items per basket” and “price per item”
Or, in other words, the Commercial Equation:
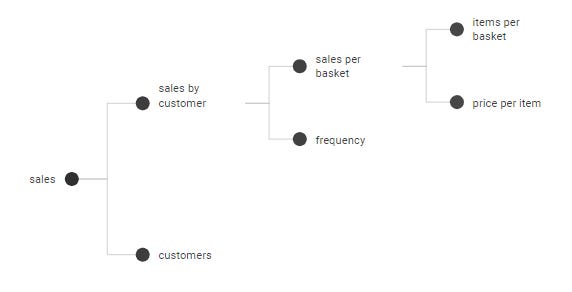
Of course, you probably saw the above metrics in some format or another.
They are deceptively simple. If you to demonstrate a hiring manager that you know about the commercial equation, you pass that interview. But to pass the interview with the analytics manager, you have to know that the framework above is actually quite complex.
The Complexity of Simple Things
The commercial equation can tell you, at a glance, what’s happening with a retailer. Are they losing or gaining customers? Are the customers buying more or less? Why more? Why less?
But the commercial equation can also tell the same thing about a single store, a product category, or a customer segment. In fact you can filter the commercial equation to almost any level or segment imaginable for, in its smallest unit, the commercial equation can show the behavior of a single customer with a single item.
On big retailers, with more than a million customers and thousand millions baskets, each one with dozens of products, you can’t calculate the commercial equation without a modern data warehouse and proper data pipelines in place.
As the number of different filter you can use grows, the calculation requirements explodes. Because it's necessary to count the number of distinct customers anew for every new filter, analytics managers need to understand how to effectively handle that data and the ecosystem to support it.
Show that analytics manager you know how to handle it, and the job’s as good as yours.
Oh, wait. what’s that? The analytics manager threw you a follow-up question? Ah, and it’s a scenario analysis? Bring it on!
“So, I have a store that’s not doing very well…”
…and he wants to know what would you do to fix it, right? Is that what they asked you? You know the answer: start with the Commercial Equation. Let’s throw it in there.
Unpacking the Commercial Equation for a single store/category/region will look pretty much like this:
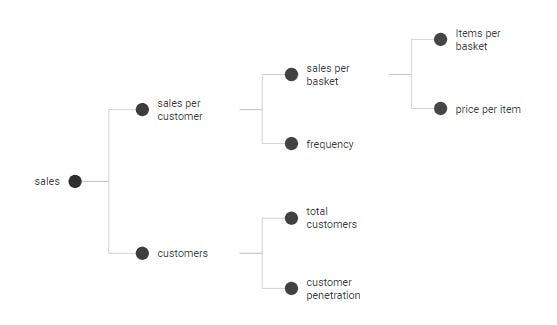
Notable new KPIs are: total customers and customer penetration.
Ask for the YTD (year to date) or QTD (quarter to date) figures for this year and the last year for comparison. They will ask you why you choose the last year. Your answer should be: “Because of seasonal effects, it makes sense to prefer year-over-year (YoY) comparisons in retail”. You can also ask your interviewer for these to be like-for-like (LFL) numbers. No, I don’t mean the Youtube practice of liking a video expecting a like in return! This is retail jargon for when you only consider the stores that were already opened in the comparison period of your analysis. That way you remove the effect of new customers coming into new stores.
It’s very likely that the analytics manager interviewing you will be seized by a fit of euphoria when they hear this.
Let’s move on. You have the figures: sales dropped. The sales per customer stayed the same, but there was a drop in the number of customers. There: you've just pinpointed the main cause.
But have customers left only that store? Or has the business as a whole shrunk? That’s what the penetration and the total customers KPIs are for. The customer penetration tells you the share of shoppers a given store has over the total numbers of shoppers in the business. A negative penetration means that store is leaking customers faster than all stores in the business combined.
A drop of customers, together with a positive customer penetration and a diminishing number of total customers, tell a different story. It means that, although the company is getting smaller, that store or category is holding its own.
PSA: Please refrain from the “ops, maybe I should be interviewing for another company then” joke! It’s not the time to check if the manager has a dark humor.
The Final Form
Obviously, you will remind your interviewer you are aware that not everyone is using a loyalty program (they nod). Show them the Complete Comercial Equation. It looks like this:
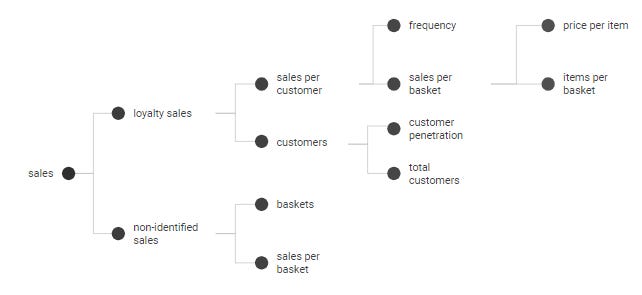
They will smile. You’re in.
But it’s not over yet.
You will get the job, yes, but the Commercial Equation is only the first analysis you should run. Depending on the problem it detects, you will have to run other analyses. In your first month of your new job, hit the ground running with the following tools:
Losing customers for a category? Launch a customer balance sheet analysis to figure out where these people are going.
Track a weekly Commercial Equation to get ahead of trends.
How did my new product performed? Or why is it doing so bad? Have a switching analysis at the ready and find out what people are buying instead.
What are the effect of a price change? Run a customer profile and launch a repeat purchase analysis.
The paths are many but remember: it all starts from that little program, which others may think is about loyalty, but you know it better: it’s about creating the data you'll be fooling around with in your newly minted analytics job!
Hi, Fernando!
Thank you for this great content! Many people like to talk about complex stuff but in fact the simple things still have its value (and complexity too!). We almost worked a few years ago and I’m very sure that I would have learned a lot from you. Keep posting!
Thanks again!